Social Research Methods
0.0 / 5
- Created by: Rabbs68
- Created on: 25-02-24 12:00
What is research design
provides a framework for the collection and analysis of data. A choice of research design
refl ects decisions about the priority being given to a range of dimensions of the research process
refl ects decisions about the priority being given to a range of dimensions of the research process
1 of 61
4 Considerations for research design
• expressing causal connections between variables;
• generalizing to larger groups of individuals than those actually forming part of the investigation;
• understanding behaviour and the meaning of that behaviour in its specifi c social context;
• having
• generalizing to larger groups of individuals than those actually forming part of the investigation;
• understanding behaviour and the meaning of that behaviour in its specifi c social context;
• having
2 of 61
What is a research method?
A research method is simply a technique for collecting data.
3 of 61
3 central criteria in Social research
reliability, replication, and validity
4 of 61
Reliability
To what extent the results of a study are repeatable. E.g. with qual research, can we establish a robust and stable measure of a concept like poverty or are results unstable?
5 of 61
Replication
Degree to which a study can be repeated. How well mapped was the initial process? (Reliability about steadiness of outcomes, replicability is about steadiness of process)
6 of 61
Validity
The integrity of a studies' conclusions. Several sub-categories of validity; Measurement validity, internal validity, external validity, ecological validity.
7 of 61
Measurement Validity
I.e. construct validity. Does the measure of the concept really reflect the concept it is meant to be denoting? E.g. does IQ really measure intelligence?
8 of 61
Internal Validity
How valid is our assumption that x causes y? How much of the variance in y is accounted for by x? (R^2 in Stats). How confident are we that the independent variable drives the dependent variable?
9 of 61
External Validity
How generalisable are the study's findings beyond the specific research context?
10 of 61
What is a Case?
A case is one row of a dataset. Cases have attributes and the measure of an attribute across multiple cases is called a variable.
11 of 61
Measuring Qualitative Research Design
Credibility (internal validity)/Transferability (external validity)/Dependability (are the findings applicable across time?)/Confirmability (objectivity)
12 of 61
Experimental Design
Can require high manipulation of complex concepts like poverty to create control groups. More manipulation risks minimizing the generalisability.
13 of 61
Classical experimental design (RCT)
The independent variable is manipulated through randomized grouping and the effect on the dependent variable is measured.
14 of 61
RCT notation
Obs = observation of dependent variable. Exp = experimental treatment. No Exp = no experimental treatment. T = timing of observation.
15 of 61
RCT: Challenges to Internal Validity (need for a control)
History: Other independent variables that could shape the change in the dependent variable. Testing: pre-tests could sensitize subject to the experiment aims. Instrumentation: changes in administration of test could generate variance in dependent variable
16 of 61
RCT: Challenges to external validity
Selection (generalisability across people)/Setting (generalisability across contexts)/History (generalisability across time?)/Pre-testing/Reactivity to experiment
17 of 61
Laboratory experiments
Higher in internal validity due to enhanced control. Diminished generalisability because of interference. Selection/setting/reactivity will interact with dep. var.
18 of 61
Quasi Experiments:
Experimental designs with concessions on internal validity. E.g. natural experiments. e.g. effects of television on children's behaviour when first introduced to a population
19 of 61
Evaluation research
Measuring the effectiveness of a programme/policy intervention. Experimental designs are popular. Alt methods like qual investigation can also be used.
20 of 61
Cross-sectional research design
Examples: surveys/structured observation/content analysis, diaries. Focus is on patterns of variation across cases at a single point in time. No time ordering, so no causal direction. Lower internal val
21 of 61
Cross-sectional: strengths/weaknesses
Good replicability with well-mapped processes. Weak internal validity (associations not causality) unless one variable is known to precede the other, e.g. race precedes alcohol consumption.
22 of 61
Longitudinal designs
Variation between cases across time. V expensive Common types: panel study (representative sample, so variables can be controlled for if sample is large enough), cohort study (sample grouped by a variable, e.g. birthd
23 of 61
Longitudinal design Problems
Sample attrition: death, loss of contact...mitigation is frequency of interactions. Large datasets collected without due planning
24 of 61
Case Study Design
Single Case; can be a person/a community (online)/ any entity. Strong focus on the setting/context
25 of 61
Deductive vs Inductive
Deductive: starts with a theory, then RQs then data collection Inductive: starts with data collection then builds upwards.
26 of 61
Case Study: Strengths vs Weaknesses
Depends on case study process and perceived importance by researcher. Qual = lower importance, Qant = higher. External validity is not the point but indictive study of trends could lead to generalisation.
27 of 61
Case Study Typology
1. Critical case = Well-developed theory --> specific case chosen 2. Extreme or unique case --> Tail cases may exhibit unique qualities for study
28 of 61
Comparative Design
Comparing two contrasting cases.
29 of 61
Comparative Design Risks
Data in both cases must be collected so as to interoperable and usable for secondary analysis. All instruments must be handled in a way that makes the findings comparable across cases.
30 of 61
Cross cultural research in Comparative Design
Can anything be generalised across cultures? How does one control for cultural differences. How does one ensure that the reactivity is the same in culturally embedded scenarios.
31 of 61
Qualitative Comparative Design (multiple case studies)
Strengths: Multiple case studies carried out together can provide better theory building than a single case study. Weaknesses: Less attention paid to detail and more to contrasts between cases.
32 of 61
Defining Causation
Successionist: ind var acting to drive dep. var. Critical realism: generative mechanisms for observed regularities
33 of 61
What is the purpose of sampling?
To find a balance between resource constraints and producing findings that are generalisable to units of interest. Representativeness is the key consideration.
34 of 61
Key Sampling Terms: Population
Units from which sample is selected.
35 of 61
Key Sampling Terms: Sample
Subset of population selected for investigation. Probability vs non-probability approach.
36 of 61
Key Sampling Terms: Sample Frame
Units of interest within population
37 of 61
Key Sampling Terms: Representative Sample
sample represents the population in microcosm
38 of 61
Key Sampling Terms: Sampling bias
Where not all members have an equal or known chance of being represented in the sample. Distortion of representativeness
39 of 61
Key Sampling Terms: Probability Sample
Each unit has a known probability of being selected. Theoretically; a representative sample is more likely through this process. Aim is to minimise sampling error.
40 of 61
Key Sampling Terms: Non-Probability Sample
Some units are more likely to be selected than others.
41 of 61
Sampling Error
Error in research findings due to difference between population and sample. Probability sampling cannot guarantee the elimination of sampling error. i
42 of 61
Non-sampling error
errors in research findings due to diff between pop and sample due to sampling approach , inadequate sample frame, non-response or poor question wording, poor interviewing, flawed data processing.
43 of 61
Census
enumeration of the entire population, data being collected in relation to all units in a population
44 of 61
Non-random Sampling; weaknesses
human bias in selection can introduce bias into the sample
45 of 61
Inadequate Sampling frame
Incomprehensive or inadequate sampling frame can make representativeness impossible by excluding units.
46 of 61
Non-response
Non-response can be problematic because the individuals who choose not to respond may have similar characteristics.
47 of 61
Probability Sampling benefits
Can minimise sampling error, produce representative sample that permits statistical inference
48 of 61
Types of probability sample
Simple random sample: pick members at random from population. Systematic sample: use a formula to pick units from the population, e.g. every 20th student in list of Student IDs. Stratified random samp
49 of 61
Stratified Random Samples
Strengths: ensures representative nature according to stratified criteria. Can reduce the standard error in sampling due to variance between strata being eliminated from each sample. I.e. if tall and short people are separately sampled for jumping ability
50 of 61
Cluster sampling
Grouping together units in a population before running a sample of some kind. E.g. sampling UK students by first clustering by university and sample 10 unis. Saves on travel time. Weakness: could o
51 of 61
Sample Size
As sample size increases, the sample error decreases; it is more likely that the representativeness of sample will increase, but no guarantee. Less tolerance for sampling error, larger required population
52 of 61
Response Rate
Usable questionnaires/ total sample (- unsuitabe or uncontactable memebers)
53 of 61
Non Probabilistic Sampling
Convenience Sampling/Snowball Sampling (case recommends next cases, emphasises networks or relationships (low likelihood external validity/representativeness)/Quota Sampling (non-randomised (unrepresentative) selection of people to make sample representat
54 of 61
Qualitative Sampling (Purposive Sampling)
Cases chosen based on relevance to research questions.
55 of 61
Qualitative Sampling Approach
Sample contexts then sample participants. Qual highlights embedding of participants in their place.
56 of 61
Qual: Theoretical Sampling
Sampling runs until theoretical saturation achieved. I.e. cases stop telling you something new about theory/hypothesis. Theoretical reflection drives need for further sampling.
57 of 61
Quote on Theoretical vs Statistical (quant) sampling
‘Theoretical sampling is done in order to discover categories and their properties and to suggest the interrelationships into a theory.
Statistical sampling is done to obtain accurate evidence
on distributions of people among categories to be used in
d
Statistical sampling is done to obtain accurate evidence
on distributions of people among categories to be used in
d
58 of 61
Purposive sampling strengths
Avoids wasted resource sampling x% of people. Focus on those who inform understanding of theory or concept.
59 of 61
Grounded theory vs Generic inductive
Hood usefully contrasts grounded theory with what she calls a ‘generic inductive qualitative model’, which is relatively open-ended and emphasizes the generation of concepts and
theories but does not entail (among other things) the
iterative style of gr
theories but does not entail (among other things) the
iterative style of gr
60 of 61
Qual Sample Size
The more comparisons required, more concepts explored, the larger the sample size. (20-30 in academia). But really it should be tailored to your research.
61 of 61
Other cards in this set
Card 2
Front
4 Considerations for research design
Back
• expressing causal connections between variables;
• generalizing to larger groups of individuals than those actually forming part of the investigation;
• understanding behaviour and the meaning of that behaviour in its specifi c social context;
• having
• generalizing to larger groups of individuals than those actually forming part of the investigation;
• understanding behaviour and the meaning of that behaviour in its specifi c social context;
• having
Card 3
Front
What is a research method?
Back
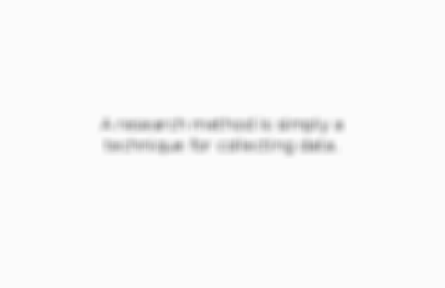
Card 4
Front
3 central criteria in Social research
Back
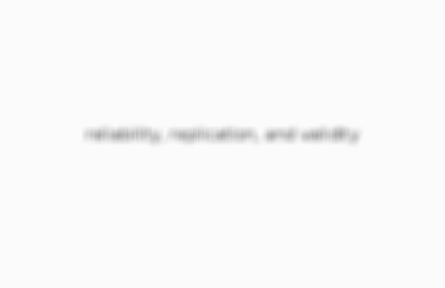
Card 5
Front
Reliability
Back
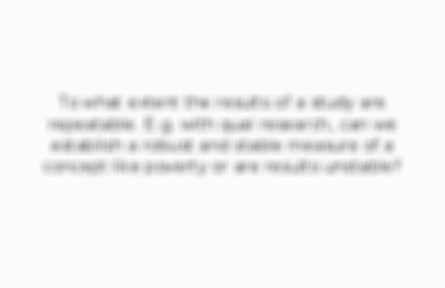
Related discussions on The Student Room
- Can I take a university degree in social research? »
- BTEC H/S Care Unit 4: Enquiries into Current Research in Health and Social Care »
- Hnc social sciences graded unit »
- MA Big Data at KCL vs MSc Social Research Methods LSE »
- Synoptic Question - Psychology AQA GCSE »
- HSPS Research Methods? »
- Unit 4 Health and Social »
- A-level OCR Sociology help! »
- Aqa a level psychology »
- Someone please help me urgently:( psychology alevel »
Similar Economics resources:
0.0 / 5
0.0 / 5
0.0 / 5
0.0 / 5
0.0 / 5
0.0 / 5
5.0 / 5 based on 1 rating
Teacher recommended
5.0 / 5 based on 1 rating
Teacher recommended
Comments
No comments have yet been made