Resampling Statistics
0.0 / 5
- Created by: francesca_321
- Created on: 27-04-17 13:44
Why use resampling techniques?
Fewer assumptions, (more accurate), very general, no equations or tables to look up and force us to think about our data
1 of 57
Why are resampling techniques not popular?
They require programming, there is a perception that new techniques must be more complex and people do not think about their data
2 of 57
What is the first general principle?
motivation for inferential statistics: to determine the probability that the differences we measure were caused by sampling error
3 of 57
What is the principle of resampling techniques?
Measure that sampling error by repeating the sampling process a large number of times:
4 of 57
What can we determine?
likely error introduce by the sampling by looking at the vulunerability in the resampling
5 of 57
How can we run resampling techniques for between subject tests?
we want to determine the likelihood of getting differences this extreme if the data all came from a single population
6 of 57
Therefore what needs to be stimulated?
running the experiment many times, with the data coming from one population; check what range of values is normal.
7 of 57
In practice, what happens to the data?
keep the measured values but shuffle them (randomly assigning them to the two groups); count how often the difference between the new means is bigger than between the measures means?
8 of 57
What do we assume about the data?
These are real and sensible values
9 of 57
What do we not assume?
Anything about their distribution
10 of 57
How many times is this process repeated?
10000
11 of 57
What is a between subject test?
our measured diff between means: 4.7, 3304 resampled differences were more extreme than 4.7, which is 33% of 10,000, 33% of values were more extreme, p=0.33 )005
12 of 57
What is the summary of between subject tests?
repeat simulated experiment a large number of times, assuming the null hypothesis is true, and check how extreme the real value was • no equation needed, except for the statistic of interest, e.g. mean • no table needed: the data gives p value
13 of 57
What happens if the hypothess differ in Standard deviation rather than the mean?
Repeat the process a large number of times, the measured difference of the Standard deviations was 8.59, 251 of the resampled diffs were more extreme than this: p= 0.025 (
14 of 57
What do we not need to do?
we do not need a whole new test if we change our opinion of what is interesting in the data • we do not need a parametric and a nonparametric version of the test • very similar approach for within-subjects design
15 of 57
What happens with a within subject randomisation test?
now the populations that we randomise are within subjects: in each resample, the values are shuffled for each subject, rather than across the whole dataset: we just randomise the sign of the difference for each pair
16 of 57
After the process has been repeated what happens next?
The measured mean difference was 0.12, 1706 (17.1%) of the resampled mean differences were more extreme p = 0.171 (> 0.05)
17 of 57
What do T tests do?
Take number of subjects into account
18 of 57
The sample size for each resample has to be the same as the original data, therefore what does the variance have to be?
mean differences will automatically reflect the number of subjects
19 of 57
Therefore?
Across a range of heights, a measured difference of 0.25ft (3 inches) would be a surprising if the group size were 100, but not if group size were 10
20 of 57
What is an addendum?
There might not always be as many as 10,000 possible ways to shuffle the original sample
21 of 57
What is it more efficient to do?
Use only the possible resamples
22 of 57
What do resampling methods measure?
sampling error by repeating the sampling process a large number of times
23 of 57
Therefore what can we determine?
likely error introduced by the sampling by looking at the variability in the resampling
24 of 57
What is the bootstrap method?
Work with single group and resample with replacement
25 of 57
What can the bootstrap resample be used to calculate?
confidence interval of a mean, standard error of the mean
26 of 57
What else can they determine?
Whether some test value is inside or outside the 95% confidence interval
27 of 57
What do the non parametric methods presume?
Make very few assumptions of the data
28 of 57
What is the first step in the bootstrap example?
Matching up data
29 of 57
What does 10,000 resampled means show?
A good approximation of possible values that the true population means is likely to exhibit
30 of 57
How can the SEM be calculated?
Standard deviation of the means of all possible samples and it can be estimated from the standard deviation of bootstrap
31 of 57
In our example what is shown?
s.e.m based on the formula (see week 4): 4.27 – s.e.m. based on the bootstrap resamples: 4.10
32 of 57
What is the difference?
the difference is due to the fact that they are both estimates, calculated in two different ways
33 of 57
What does calculating a confidence interval represent?
the range of values that 95% of the means take
34 of 57
How is it calculated?
From the bootstrapped means by ordering them and cutting off the highest and lowest 2.5%
35 of 57
for example of a one sample test?
let us consider the hypothesis that this population is „above average IQ‟. Then we might expect: IQ>100
36 of 57
What do we want to know?
Whether the mean IQ is greater than 100
37 of 57
Therefore if H0 was true, how likely was this data?
How likely is a mean of 100 or less for our population
38 of 57
What can we do?
Count how often it occurs within the bootstraps, order and find how many values are
39 of 57
What does bootstrap actually mean?
comparing the mean to a specific value is effectively having a very simple model of the world • bootstrapping generalises easily to more complex models than just the mean
40 of 57
Therefore what could you do?
Draw a line of best fit and work out the gradient
41 of 57
What is the bootstrap approach to confidence intervals?
take bootstrap resamples of the subjects, the resamples can be made within experimental conditions, or across the whole dataset: choose the way that matches the experiment in our smoking smurf experiment, use a resample within each condition
42 of 57
What does Bootstrapping confidence intervals resampling allow?
resampling within the conditions constrains the simulations so that there are always 10 subjects per condition
43 of 57
What is the second step in bootstapping confidence intervals?
.for each resample, perform the analysis (e.g. measure the gradient of the line of best fit)
44 of 57
What is the last step in bootstrapping confidence levels?
.percentiles (or some other measure of spread) can be taken directly from the bootstrap distribution of the statistic
45 of 57
Therefore what do you get?
the 2.5 and 97.5 percentiles of the gradient which is 95% confidence interval
46 of 57
What happens in more complex bootstrapping, you have to draw a curve
, you have to draw a curve
47 of 57
What is the equation?
y=Xn/Xn+Kn
48 of 57
how do you find the subjects threshold?
Find the value where the subject is at some criterion eg 80%
49 of 57
What happens if you want to know the confidence interval for the threshold?
Resample the data using bootstrap method • For each resample, fit the curve again, take the new threshold value and repeat for each sample, continue as in previous methods
50 of 57
What are the advantages of bootstrapping?
very general method: any type of model can be used and confidence intervals on any of its parameters can be estimated • can also be used to perform hypothesis testing (especially for one-sample tests)
51 of 57
What other advantages are there?
not based on any assumptions about the data • no tables, no equations (except for the model)
52 of 57
What are 2 issues with bootstrap?
How many data samples (n) do I need? no a priori answer, try and see. • How many resamples must I generate? 1,000 – 10,000 depending on how accurate you want your p
53 of 57
What are other 2 issues with bootstrap?
Which type of resampling should I use? whatever best simulates the original sampling • What if my data are not representative of the population? garbage in garbage out
54 of 57
What is meant by garbage in, garbage out?
Computing and other fields can often be incorrect or poor quality which produce faulty output
55 of 57
What is the Jack Knife resampling technique?
similar to bootstrap but rather than „randomly sampling with replacement‟, resampling is done by „selecting all data except one
56 of 57
What is the montecarlo method?
create data based on model simulations and compare these to real data e.g. if a neuron has a certain spike rate and a „Poisson‟ spike generating mechanism what is the chance of seeing a particular pattern of spikes
57 of 57
Other cards in this set
Card 2
Front
Why are resampling techniques not popular?
Back
They require programming, there is a perception that new techniques must be more complex and people do not think about their data
Card 3
Front
What is the first general principle?
Back
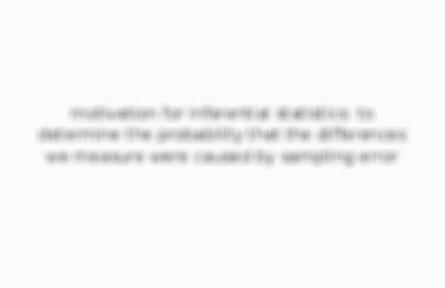
Card 4
Front
What is the principle of resampling techniques?
Back
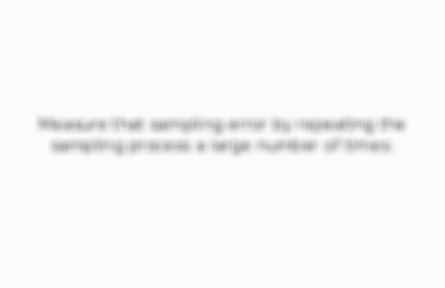
Card 5
Front
What can we determine?
Back
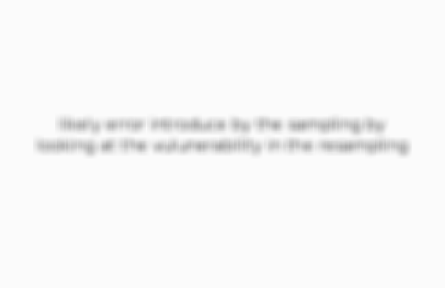
Related discussions on The Student Room
- Is Undergrad Psychology hard? »
- Academic literacy »
- GCSE Edexcel Statistics resources »
- A level maths hypothesis tests »
- Advice on personal statement for Statistics and Philosophy? »
- Psychology undergrad »
- quant finance »
- MSc Statistics online/distance learning »
- Economics and Maths »
- MSc Statistics Part Time »
Similar Psychology resources:
0.0 / 5
5.0 / 5 based on 1 rating
0.0 / 5
0.0 / 5
0.0 / 5
Comments
No comments have yet been made